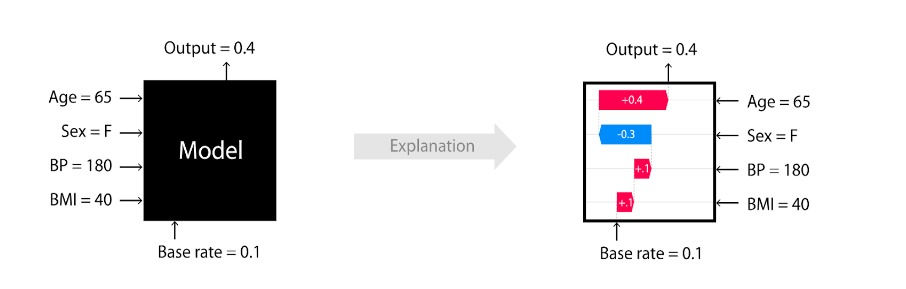
As the field of artificial intelligence continues to expand, so does the need for understanding how these complex models arrive at their decisions. The need for explainability is not only important for legal and ethical reasons, but it also helps in building trust in the model and making informed decisions. The Shapley Additive Explanations (SHAP) method is a powerful technique that provides a unified framework for interpreting any model. In this blog post, I will explain the SHAP method and how it can be applied to interpret machine learning models.
Understanding SHAP: A Game-Theoretic Approach
At its core, SHAP operates on the principles of game theory. Just as game theory calculates the contributions of individual players to a collective payout, SHAP calculates the influence of each feature on the final prediction of a machine learning model. Imagine a strategic game where each player's contribution is carefully evaluated to determine their impact on the game's outcome. In SHAP's world, these players are features, and the payout corresponds to the model's prediction.
SHAP's ultimate goal is to assign a score to each feature within a machine learning model. This score represents the weight of the feature in determining the model's output. However, in the field of complex models with numerous interplaying features, calculating these scores can become exponentially complex. To tackle this challenge, an approximation method called Kernel SHAP comes to the rescue.
How to apply SHAP?
To apply the SHAP method, we need to first compute the Shapley values(concept popularly used in Game Theory) for each feature in the input space. This can be done using one of the many implementations available in popular machine learning libraries, such as scikit-learn, XGBoost, and TensorFlow. Once we have the Shapley values, we can visualize them using various techniques to gain insights into the model's decision-making process.
One popular technique to visualize the Shapley values is the Shapley value plot, which shows the contribution of each feature towards the model output for each individual data point. The length of the bar represents the magnitude of the Shapley value for the corresponding feature. The color of the bar represents the value of the feature, where red represents high feature values and blue represents low feature values. The plot helps in identifying the most important features for each data point and the direction of the relationship between the features and the output.
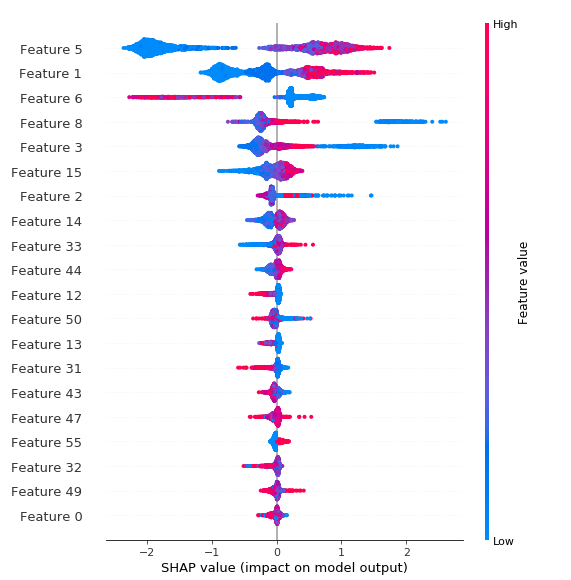
Another technique to visualize the Shapley values is the summary plot, which shows the average contribution of each feature across all data points. The plot consists of a horizontal axis representing the Shapley value and a vertical axis representing the features. Each feature is represented by a horizontal bar, where the length of the bar represents the magnitude of the average Shapley value. The color of the bar represents the direction of the relationship between the feature and the output, where red represents a positive relationship and blue represents a negative relationship.
In addition to visualizing the Shapley values, the SHAP method can also be used to identify instances where the model makes biased or unfair decisions. The method can be used to quantify the extent to which each feature contributes to the model's bias towards a certain group or class. This helps in identifying the root cause of the bias and taking corrective measures to ensure fairness and equity in the model's decisions.
Exploring the Landscape: Local and Global Explanations
SHAP's utility shines both on a micro and macro level. It can provide explanations for individual predictions (local explanations) or reveal the model's behavior across its entire range (global explanations). This versatility makes SHAP an essential tool for various domains, from healthcare to finance.
The Model Dependency Problem
One key consideration when applying SHAP method is its model dependency. The explanations SHAP provides are based on the specific machine learning model used. Different models can yield different explainability scores, and the features highlighted as influential may vary between models.
Tackling Biases and Assumptions
While SHAP offers valuable insights, it's not immune to the issues of biased classifiers. There's a possibility that SHAP-generated explanations might miss underlying biases within the model, leading to unrealistic explanations. Additionally, SHAP's assumption of feature independence can lead to skewed results when dealing with correlated features.
Ensuring Stability in the Face of Collinearity
Collinearity – the correlation between features – can introduce instability in SHAP-generated insights. Features that are highly correlated might be assigned lower scores, despite their significant association with the outcome. To address this, a method based on normalized movement rate (NMR) can assess the stability of informative feature lists generated by SHAP.
Conclusion
The Shapley Additive Explanations (SHAP) method provides a powerful framework for interpreting any machine learning model. The method is based on the concept of Shapley values, which provides a fair way to distribute the gain of a cooperative game among its players. The SHAP method provides an efficient way to compute the Shapley values for each feature in the input space, which gives us a measure of the contribution of each feature towards the model output. The method can be applied to visualize the Shapley values, identify the most important features, and quantify the model's bias towards certain groups or classes. By providing a unified framework for interpretability, the SHAP method helps in building trust in the model and making informed decisions.
If you have questions and if you’d like to reach out to us, you can find us here - [trustycoreinfo@trustycore.com]. Also, leave a comment below and let's continue this conversation here.
Comments