TrustyCore provides multiple features for AI Explainability
In order to ensure transparency, governance, and accountability in AI decision-making, TrustyCore Build of TrustyAI provides an enterprise-ready distribution of TrustyAI, an open source AI Explainability (XAI) Toolkit.
TrustyAI uses the ODH governance model and Code of Conduct.
Key Features
TrustyCore Build of TrustyAI, an open source initiative designed to infuse clarity into complex AI models, employs a range of explainable AI techniques.
​
TrustyAI core library have several tools, which includes:
-
Local explainers
-
Global explainers
-
Fairness metrics
-
ModelMesh integration
Algorithm Supported
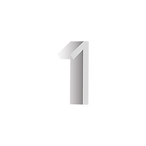
LIME(Local Interpretable Model-Agnostic Explanations)
TrustyAI incorporates LIME, a method that helps you understand “What really happened” to the prediction when adding perturbed input into the model.
PDPs(Partial Dependence Plot)
Helps to observe how changes in a certain feature influences the prediction, on average, when all other features are left fixed.

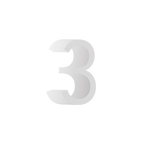
SHAP(SHapley Additive exPlanations)
Assigns each feature an importance value for a particular prediction.
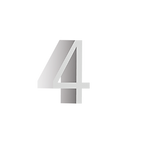
Counterfactual Explanation
Provide users with alternate scenarios where outcomes would change.
What we offer:
TrustyCore AI Explainability
TrustyCore AI Explainability is built on the TrustyCore Build of TrustyAI, providing xAI for AI/ML decisions. Available as a SaaS or as an on premise / private cloud deployment.
TrustyCore Dashboard
TrustyCore Dashboard allows enterprises to implement a work dashboard for human review of AI/ML decisions that are high risk, and integrates TrustyCore Explainability into the interface to simplify human processing.
TrustyCore Risk Filter (Coming Soon)
TrustyCore Rules allows enterprises to define which AI/ML decisions may be high risk allowing government regulations, industry requirements, or internal policies to be monitored for explainability.